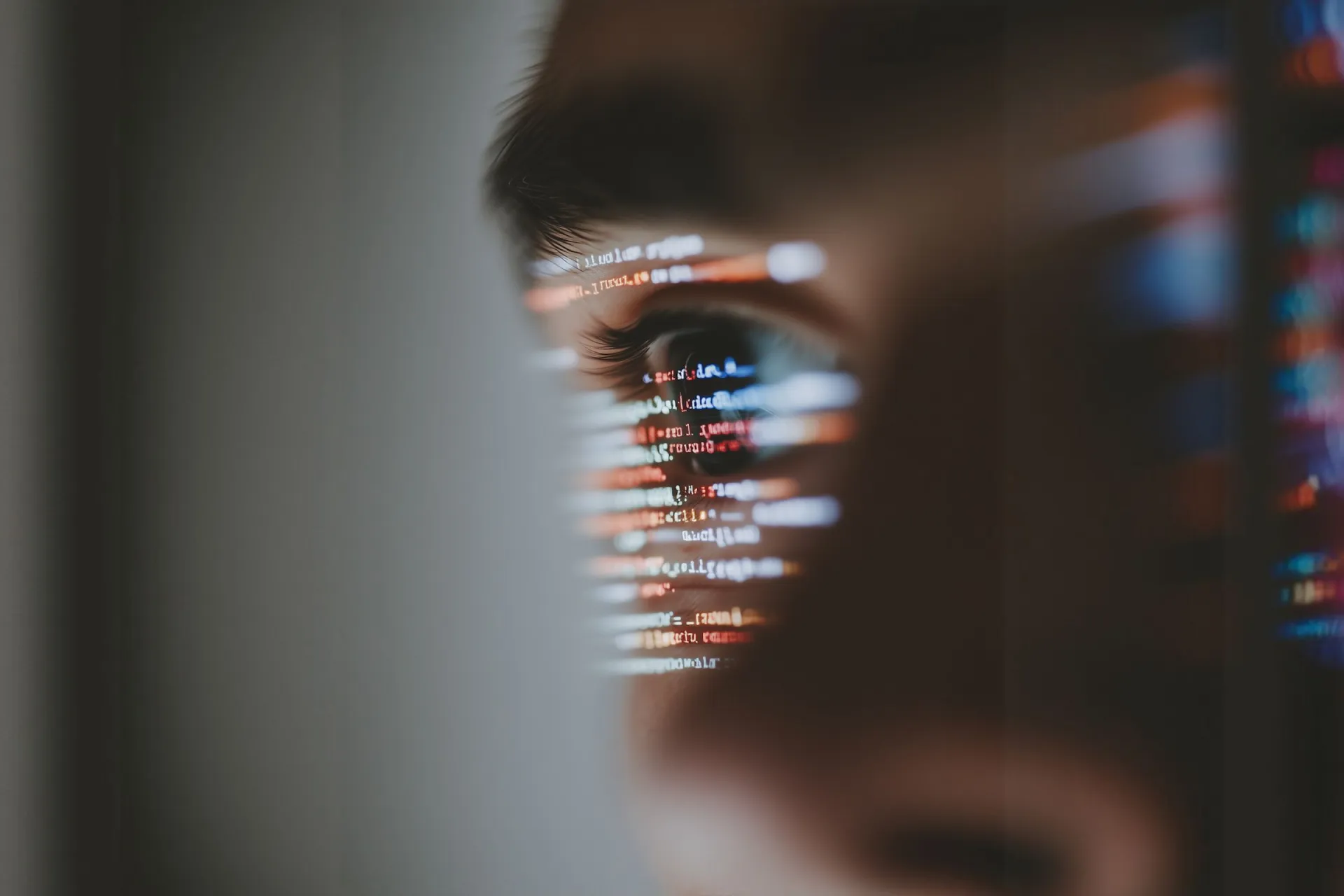
In traditional science, data followed discovery. You ran the experiment, then analyzed the output. Now, data is baked into the beginning. With machine learning and computational biology, researchers can simulate outcomes, stress-test hypotheses, and refine models before a single sample is touched. Discovery is no longer linear—it's iterative, intelligent, and faster than ever before.
Genomics and synthetic biology are generating vast, complex datasets that describe life at its most fundamental layers. AI tools are turning that data into dynamic insight—predicting protein behavior, modeling disease pathways, or even designing new biological components. We're not just interpreting the genome. We're starting to speak its language.
Human capability doesn't exist in isolation—it's embedded in systems: health systems, learning environments, operational frameworks. Data allows us to understand those systems with extraordinary granularity. From hospital networks to neural networks, we can now diagnose systemic inefficiencies, model solutions, and implement change with precision. We are approaching a point where data doesn't just inform decisions—it actively shapes outcomes. It enables personalized therapies, real-time diagnostics, and adaptive systems that respond to human behavior. The question is no longer what can we measure?—it's how will we choose to use what we know?
"We've gone from decoding life to designing it—and data is the scaffolding holding that future together."
Data once followed the experiment. Now, it defines the hypothesis. Machine learning models can simulate outcomes before physical trials begin, compressing what once took months into days. This shift doesn't just increase speed—it alters the nature of discovery itself.
Scientific progress is no longer confined to labs and research centers. It's embedded in code, in infrastructure, in intelligent systems that can learn, adapt, and improve without direct intervention. We've moved from observing reality to orchestrating it. Data once followed the experiment. Now, it defines the hypothesis. Machine learning models can simulate outcomes before physical trials begin, compressing what once took months into days. This shift doesn't just increase speed—it alters the nature of discovery itself.
That scaffolding is already supporting some of the most advanced breakthroughs in human health and capability.
These aren't speculative ideas. They're active systems in motion, rewriting the rules of what's biologically possible.